Knowledge-Pushed Choice Making typically supplies an ideal reply to the “Flawed Query”.

These days, it’s onerous to seek out any agency that doesn’t put “Knowledge-Pushed Selections Making” (DDDM in brief) on the highest of their technique record. Corporations have extra information than ever, and their pure incentive is to learn the way they’ll create worth out of this information. Nonetheless, in line with the report¹ (survey of 190 executives throughout varied industries within the U.S.):
Solely 32% of firms reported having the ability to understand tangible and measurable worth from information.
Right here, I want to provide an perception that will clarify why firms can’t obtain the worth from the information. You will need to acknowledge that, in DDDM, the main target level is the information. We hear quite a bit in CEO talks, firm displays, medium articles :), and so on., phrases like “Knowledge has a greater thought” or “Knowledge is the brand new gold”. The primary thought of “Knowledge-Pushed Selections Making” boils right down to:
What Perception can we extract from the information?
I wish to inform you that following the above query typically results in asking “Flawed Questions”. Within the DDDM method, we attempt to discover a objective for the information at hand. Nonetheless, that isn’t the purpose of decision-makers. The purpose of decision-makers in firms is to create worth, which solely comes by way of “Excessive-High quality” selections. In organizations, we have to discover the related information that serves a objective for the selections.
The one means organizations can create worth is thru making high-quality selections, and the information must serve to attain that objective.
With this background, I want to undergo an instance of a enterprise resolution, the place first I contemplate the case of the “Knowledge-Pushed” method. It should comply with with one other different to repair some flaws within the method by way of the “Choice-Pushed Knowledge Analytics” method.
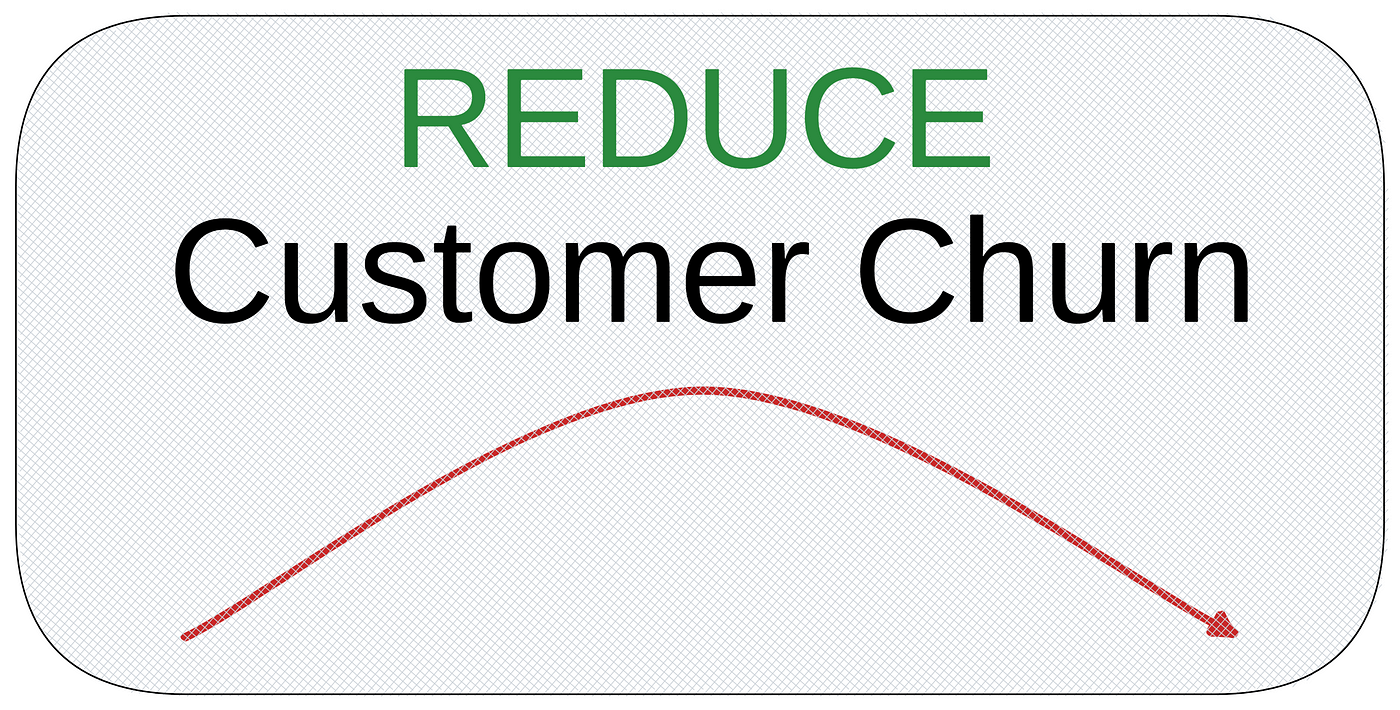
For any SaaS (Software program as a service firm) which serves a product to its buyer, churn analytics performs an necessary position. To place it merely, churn analytics entails constructing a :
A predictive churn mannequin that appears on the person exercise from the previous and based mostly on buyer options then creates a mannequin that offers the chance of costumer is ending your service or product.
We as prospects all could churn from a specific service by way of the²:
- Ending the subscription
- Closing the service account
- Refuse to resume a contract or service settlement
And the SaaS firm wants to seek out options to scale back the churn charge, as³
Buying a brand new buyer is 6–7 occasions extra expensive than retaining an previous buyer.
Given this temporary description of the case, we comply with the DDDM method to determine for this drawback:
Knowledge-Pushed Method:
Now, let’s undergo one instance of creating a machine studying (ML) mannequin that can provide us the chance the client will go away the subscription (churn chance). The ML mannequin is predicated on the in-house information of the corporate. This ML mannequin will then be used to “Resolve” for which buyer a Present card (10$) is distributed when the subscription of the individual ends (to resume the subscription, the corporate has an annual charge of 100$). For instance, under is the previous person information of an “On-line Buying Web site.” The person named “David” (26 years previous) has had his final buy 19 days in the past and has had a login to his account as soon as throughout the earlier month.

Within the above desk, we see the “Knowledge” of the previous prospects, the place the column title “Churn” says whether or not they churned (stopped the subscription) the service (subscription right here) (Churn=1) or didn’t (Churn=0).
Now, given this information, we are able to construct a machine studying mannequin that claims, for any individual sooner or later (unseen information), what chance will the individual churn?
To place it from a easy ML perspective,
X: Column of options (Orange columns)
Y: Column of output (Inexperienced column)
Having constructed a “Predictive Mannequin”, now think about the “On-line Buying Web site” must determine for 3 prospects that their subscription finish quickly, ship a present card to keep away from “Churn” of the individual. (They will ship a ten$ Present card just for one individual).
Now the resolution to make is:
Amongst three prospects (James, Mohammad, and Helen) ending their subscription quickly, which one do we have to ship “Present Card”, to scale back the churn charge?
We use our ML mannequin to make this resolution, apply it to a few prospects, and ask ML to foretell, “What’s the chance the individual will churn”?
Within the under desk, you see the ML mannequin predicted that James has an 80% chance of ending the subscription, Mohammad 60%, and Helen 40%.
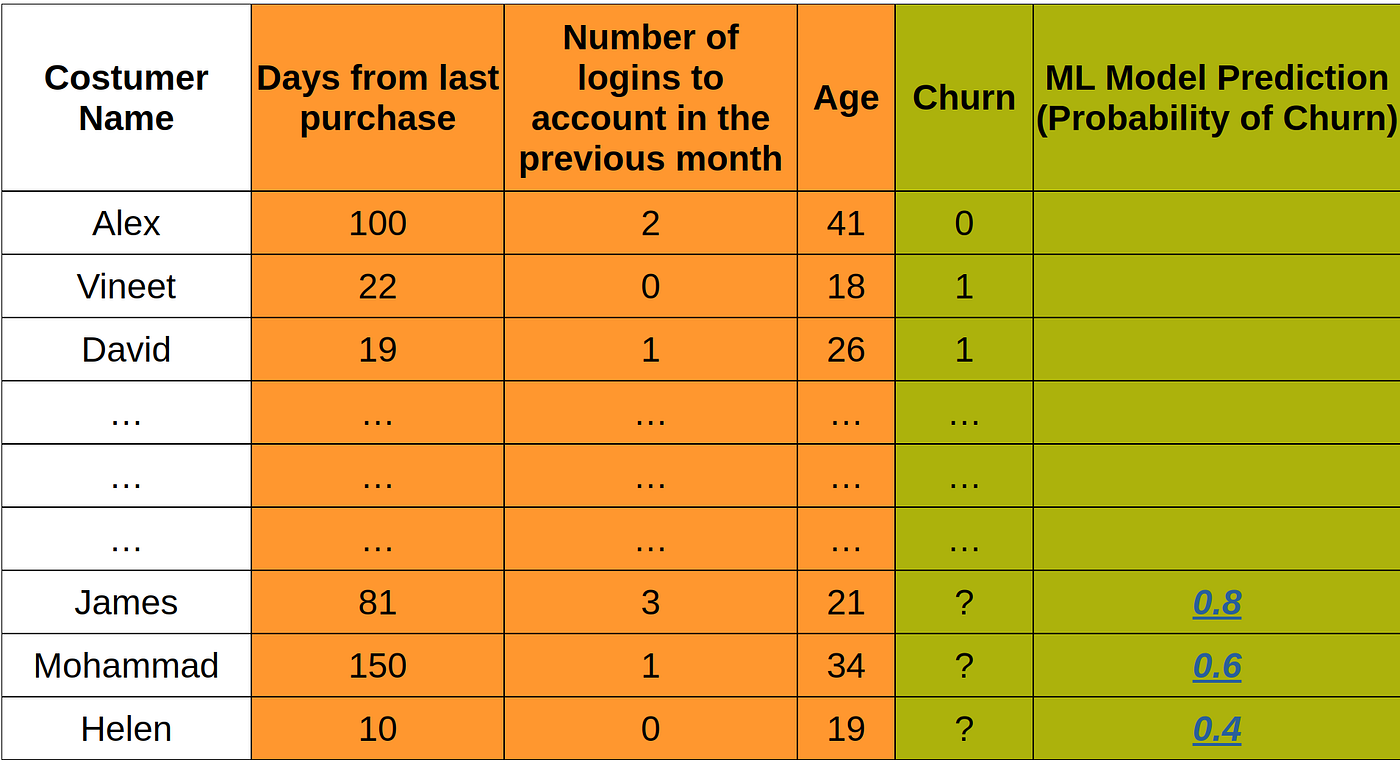
Given the prediction of the ML mannequin, it may be justifiable that the “Present Card” must be despatched to James, as he’s the client with the most important chance of leaving the subscription. I feel this can be a logical resolution to make, as we attempt to ship the “Present Card” to the individual with the best threat of leaving the subscription.
Nonetheless, as I’ll define under, the above method has some flaws (although seems rational) and results in making a “suboptimal” resolution.
Choice-Pushed Method:
Right here, on this part, I wish to reframe the identical enterprise drawback by bringing the “resolution” in hand first after which gathering the information. On this method, the “Choice” comes first, and the related “Knowledge” to help the perfect resolution comes afterward.
As a basic resolution evaluation train, for any “resolution” making course of, we want three parts (at the least), on this case:
Right here the alternatives are whether or not to ship a ten$ Present card to James, Mohammad, or Helen (three options).
The target is to maximise the worth for the group (right here, given the price of a ten$ reward card and a return of 100$ for persevering with subscription)
We want the knowledge to assign the chance of whether or not our buyer will finish a subscription or proceed. Given these three parts, the “Choice Tree” of the issue at hand might be drawn as under:
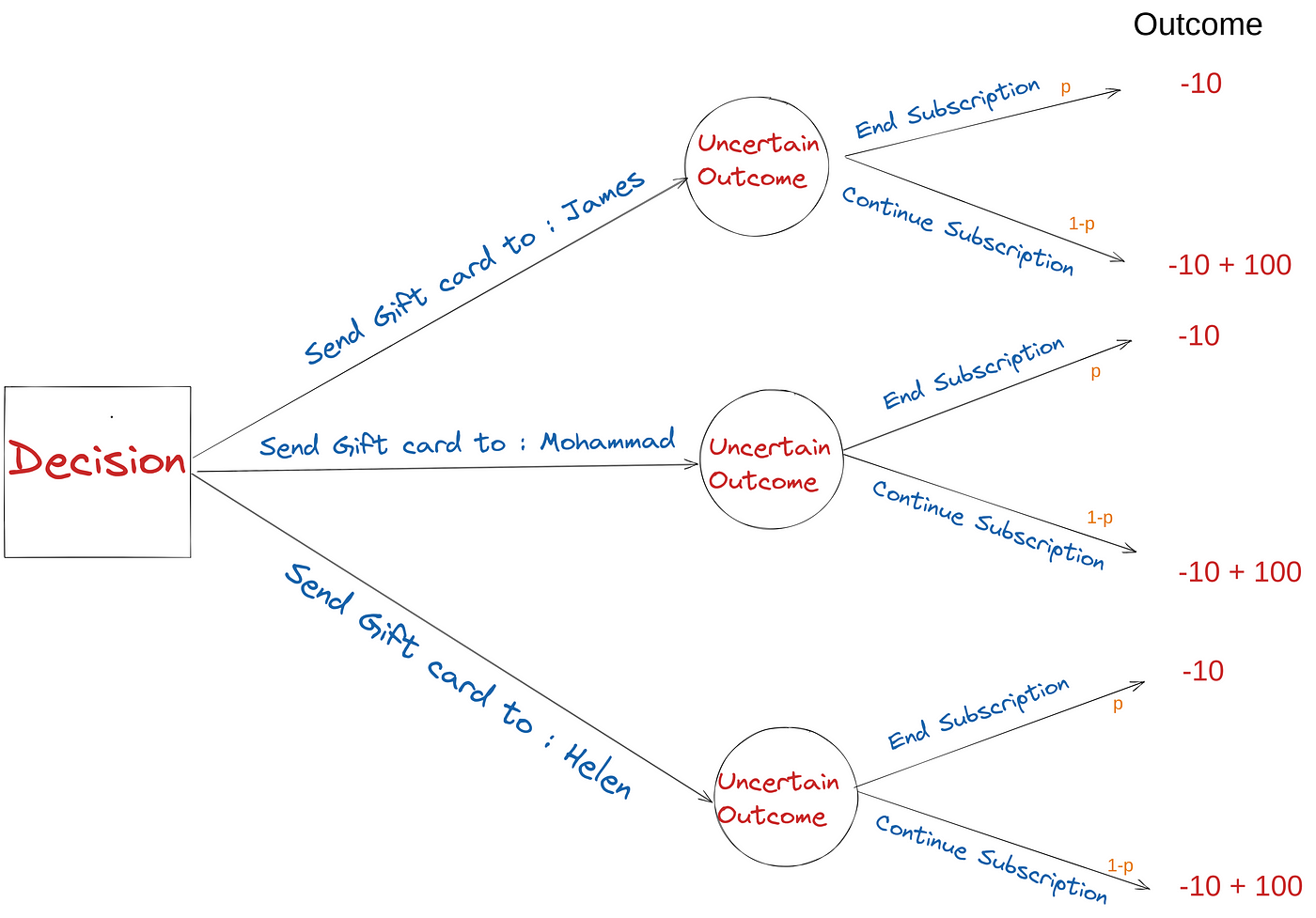
Given options, targets, and knowledge, the above resolution tree is a place to begin for framing the choice context. The Choice-driven framework asks for the related information wanted for high-quality selections. On this case, the related information is:
What’s the buyer’s chance of ending the subscription, given she is obtainable the ten$ Present card?
Value to say that, The knowledge we want within the Choice-Pushed method is completely different from the one within the Knowledge-Pushed method. Within the Choice-Pushed method, the correct information for this drawback would be the data that offers estimates of the “p” within the above resolution tree.
Organizations can purchase such data by way of experimentation, just like the A/B assessments. What you are able to do is have 100 variety of buyer and randomly give half of them the reward card (remedy)and the opposite with out andy reward card (management) and examine the “impact” of the choice. Within the chart under, I’m exhibiting the determine of a “nice” analysis paper that addresses this evaluation. The x-axis exhibits the client with the “highest” threat of leaving the subscription (R10) towards the least (R1). In our case, we are able to assume James belongs to a bunch of R8, Mohammad to R4, and Helen to R2.
Surprisingly, we are able to see that in line with the examine, the chance of ending a subscription is sort of equal to the chance of ending a subscription given by giving a present card in a renewal letter:
P(Finish Subscription) ≈ P(Finish Subscription|Receiving Present Card)

Having this data, now we are able to write down the choice tree, this time with the chance discovered from the above evaluation.

Now, having the choice outlined, the knowledge that gives an evaluation of the uncertainty and goal, we are able to decide based mostly on the “Anticipated Final result” of every selection:
Various 1) Ship a Present card to James:
EV = 0.8×(-10) + 0.2×(90) = 10
Various 2) Ship a Present card to Mohammad:
EV = 0.6×(-10) + 0.4×(90) = 30
Various 3) Ship a Present card to Helen:
EV = 0.2×(-10) + 0.8×(90) = 54
If we comply with the “Choice-Pushed” method, the reward card needs to be despatched to Helen, whereas within the “Knowledge-Pushed” method, the individual was James. So What modified that we ended up having two completely different actions to make, relying on the method,
The Knowledge-Pushed method supplies an ideal reply to the Flawed Query. Choice -Pushed Method begins with a “Proper Query” and tries to make the most of the related information to reply the query.
Take-Aways Messages:
- The purpose of the decision-maker within the enterprise, guide offering service to a consumer a, and workers who make operational selections are to create worth, and the worth solely comes by way of “Excessive-High quality” selections.
- To make the “Excessive-High quality” selections, we want first to border the choice and acknowledge its parts (options, goal, and uncertainty) and make selections based mostly on the selection with the best anticipated reward.
- The “Choice-Pushed” method supplies a framework for making “Excessive-High quality” selections. “Knowledge-Pushed” decision-making can result in a suboptimal resolution by discovering the aim for the information slightly than becoming the information to the aim.