STATISTICS
A Information to Price Implications of Making Statistical Errors for Knowledge Scientists
While you have been a baby you could have learn the story “The Boy Who Cried Wolf”. That is the story of a shepherd who used to lift false alarms about seeing a wolf and calling folks for assist when in reality there was no wolf. He repeatedly did it for his amusement, however when there was truly a wolf, and he cried for assist no person got here as a result of the villagers thought that he was mendacity once more. This can be a in style story learn to kids, particularly in ethical training and ethics courses, the place they’re informed to be trustworthy and never lie because the world doesn’t consider in liars.

People don’t at all times lie, generally there are simply errors in judgment and measurement which can have severe penalties and have the potential of adjusting companies, societies, and circumstances. There are plenty of sensible and informative sources on-line that specify intimately what the several types of statistical errors are, what the causes of these errors are, and the way to stop these errors. Nonetheless, each choice one makes in a enterprise has implications. On this article, I clarify the way to verify the price implications of creating statistical errors.
This text is printed within the following method. We are going to briefly cowl the forms of errors in statistics with some examples. After that, we outline some phrases related to the sector of error evaluation in Machine Studying: Precision, Recall, and Specificity. Thereafter, we are going to discover ways to resolve which error is extra dangerous to your enterprise by taking its financial prices into consideration. We are going to finish the article by discussing varied methods of lowering errors. This can be a common article geared extra towards the practitioners and/or newbie knowledge scientists, so I keep away from utilizing mathematical terminologies and statistical symbols. In case you’ve gotten any questions, I’m out there for a dialogue. So, with out additional delay, let’s get began.
The very first thing we have to perceive is the time period error. An error is the distinction between an precise worth and ascertained/collected worth of a amount. The upper the error is, the decrease our estimate is of the fact. For instance, if the precise temperature outdoors is 21 C, however my machine says it’s 20 C, then there’s a distinction of 1 C or a deviation of 4.76% from the precise worth. Whether or not we’re okay with this error depends upon what we’re going to do with this measurement and what the enterprise value of an inaccurate measurement can be. One of many objectives of statistical optimization is to scale back the error of our mannequin below varied contexts in order that it’s sturdy sufficient for use in a lot of eventualities.
Sort I Error, often known as False Optimistic Error, occurs when one thing is definitely not true or not current however is ascertained as true or current. For instance, if I get a COVID-test accomplished, then Sort I Error (False Optimistic Error) can be figuring out me as constructive, when in reality I’m not constructive. One other instance is the occasion of elevating a fireplace alarm when there isn’t a fireplace, which is elevating a false alarm. Another instance is convicting somebody as a prison, when in reality they don’t seem to be a prison or testing somebody as pregnant when they don’t seem to be pregnant. These are all examples of False Positives.
Sort II Error, often known as False Unfavorable Error, occurs when one thing is definitely true or current however is ascertained as not true or not current. For instance, if I get a COVID-test accomplished, then Sort II Error (False Unfavorable Error) can be figuring out me as damaging, when in reality I’m not damaging. One other instance isn’t elevating a fireplace alarm when there’s truly a fireplace. Another instance is acquitting somebody, when in reality they’re truly a prison or testing somebody as not pregnant when they’re truly pregnant. These are all examples of False Negatives.
The earlier examples present that defining one thing as false constructive or false damaging depends upon how “being constructive” is outlined in that scenario. For instance, if the occasion of seeing fireplace is seen as constructive, then a false constructive can be elevating a false alarm when there isn’t a fireplace. Nonetheless, if the occasion of not seeing fireplace is seen as constructive, then a false constructive can be seeing a fireplace. For sensible functions and ease in understanding, the taking place of an occasion is taken into account constructive. So, seeing a fireplace is taken into account constructive.
Now that you’ve got a good understanding of the phrases false constructive and false damaging, allow us to perceive three extra phrases earlier than we get to the financial prices of statistical errors. These phrases are vital from the standpoint of utilized machine studying for enterprise functions. Like earlier, we are going to overview these ideas utilizing examples from real-world life.
Suppose you’re employed for a monetary group the place the speed of curiosity provided to an applicant depends upon their previous credit score profile. An individual with a better default chance can be provided a better charge of curiosity and an individual with a decrease default chance can be provided a decrease charge of curiosity. For sake of simplicity, let’s say you’ve gotten been requested to make a mannequin that classifies the candidates into high-risk or low-risk debtors. As a result of we’ve got lots of people who don’t default, and just some who default, we can have an imbalanced dataset. Due to this fact, we can not charge our mannequin’s efficiency, simply on the premise of accuracy, thus we’d like extra metrics to charge the efficiency.
For this instance, we are going to contemplate defaulting as constructive. Thus an individual who defaults can be thought of constructive. We are going to outline and perceive recall or sensitivity, specificity, and precision now.
Sensitivity or recall is a measure of how effectively a mannequin can establish true positives, that’s high-risk debtors. In our instance, true constructive represents the mannequin figuring out debtors who will default and thus giving them a high-risk label in order that their charge of curiosity is larger as in comparison with those that have a decrease threat of default. If our mannequin’s sensitivity isn’t excessive, this may translate to extra folks getting a low-risk ranking, thus a decrease charge of curiosity, because the mannequin will be unable to discern the true positives, that’s to say, figuring out extra folks can be categorized as low-risk. Financially talking, this may be harmful for the enterprise, as extra folks will default which the mannequin predicted to be a lower-risk borrower. Thus, on this case, our mannequin ought to have a excessive sensitivity.
Specificity is a measure of how effectively a mannequin can establish true negatives, that’s low-risk debtors. In our instance, true damaging represents the mannequin figuring out debtors who won’t default and thus giving them a low-risk label, in order that their charge of curiosity is decrease as in comparison with those that have a better threat of default. If our mannequin’s specificity isn’t excessive, this may translate to extra folks getting a better charge of curiosity, because the mannequin will be unable to discern the true negatives, that’s to say, figuring out extra folks can be categorized as high-risk. Financially talking, this may be good as extra revenue can be there for the financial institution, however this may increasingly additionally imply that some debtors won’t borrow as the price of borrowing can be too excessive, which can translate to the enterprise dropping prospects or prospects getting pissed off and shifting to opponents. With knowledge one will be capable to establish which can be costlier, dropping prospects (dropping potential revenue) or making extra money from present prospects.
Precision is a measure of discernment of the mannequin. In different phrases, in case your mannequin identifies 500 folks as those that have a excessive threat of default, however actually, solely 400 persons are those that defaulted (previous knowledge), then the precision of the mannequin is 400/500 = 0.8
At this level, it’s value mentioning that discussing precision, recall, or specificity in isolation isn’t useful. You must know all three values earlier than you can also make an estimate of how expensive the measure is.
There are two steps concerned in assessing the price of making statistical errors. The primary is defining what you’ll name constructive. For instance, in our earlier instance, an individual defaulting is taken into account constructive. The second step is calculating the false constructive charge (false alarm charge), and the false-negative charge (miss charge). If the false-positive charge is excessive, which means lots of people are being rated as high-risk, which isn’t good for the enterprise. If the false-negative charge is excessive, which means lots of people are thought of low-risk, which in flip makes companies lose cash. Suppose the false constructive charge of our mannequin is 0.2, which means amongst 100 folks, our mannequin identifies 20 folks as false constructive, thus 20 prospects can be pissed off by being labeled as doable high-risk debtors. However, if the false-negative charge of our mannequin is 0.15, this may imply 15 folks out of 100 who needs to be high-risk persons are categorized as low-risk folks, thus doubtlessly inflicting the enterprise to lose cash. If a mean defaulter prices the enterprise INR 500K, then the price of the false-negative charge can be 0.15X500000 = 75000 per wrongly recognized buyer. To calculate the false constructive charge and false-negative charge, it is strongly recommended to search out the confusion matrix, and calculate the related values.
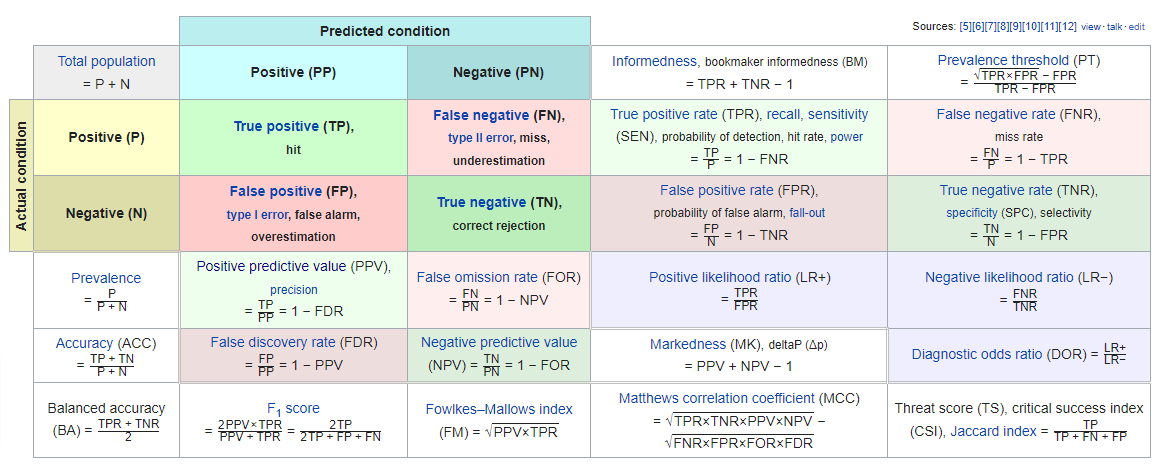
You possibly can by no means eradicate all of the statistical errors. There are methods to scale back them. These are methods you should use to scale back statistical errors.
- The Sort II Error has an inverse relationship with the ability of a statistical take a look at or mannequin. The facility of a statistical take a look at could be outlined as its true means to discern actuality and thus reject the null speculation appropriately. Which means the upper the ability of a statistical take a look at, the decrease the chance of committing Sort II Error. Thus, you possibly can enhance the ability of the take a look at. This could have value implications as higher energy results in larger pattern dimension and consequently larger prices. Lowering Sort II Error results in a rise in committing the Sort I Error. Thus, you must assess the price influence of creating these errors.
- One of many methods of lowering Sort I Error is to reduce the importance stage (the chance of rejecting the null speculation when it’s true). For the reason that significance stage is chosen by a researcher, the extent could be modified. For instance, the importance stage could be minimized to 1% (0.01). This means that there’s a 1% chance of incorrectly rejecting the null speculation (the speculation that there isn’t a distinction between two variables).
On this article, we mentioned varied forms of errors, their causes, value implications, and methods of lowering these errors. My perception is that it’s going to assist you to to make an knowledgeable choice concerning value estimation of varied forms of errors. Furthermore, it’s crucial to grasp that statistical errors can solely be minimized and never eradicated totally. One of the simplest ways to take care of the errors is to maintain engaged on their discount and have extra checks and balances (for instance, insurance coverage) to scale back the influence of creating a false judgment.