A beginner-friendly primer on two basic knowledge science ideas
Relies upon the place you’re listening to the phrase. I’ve made a tongue-in-cheek laundry record of assorted bias usages to your amusement, however on this article, we’ll deal with one particular species of bias — statistical bias —which I’ll clarify in a second.
Seize your bow and arrows! Or darts. Or cat-cannon. In case you’re a superb shot and also you’re aiming on the bullseye, what’s your goal going to seem like after you’re carried out with it? One thing like this?

Precisely.
Now think about that your purpose is biased. What’s going to your goal seem like?
Dots, dots in all places? Nope. That’s what we imply by excessive variance, not excessive bias.
As an alternative, right here’s what bias seems to be like:
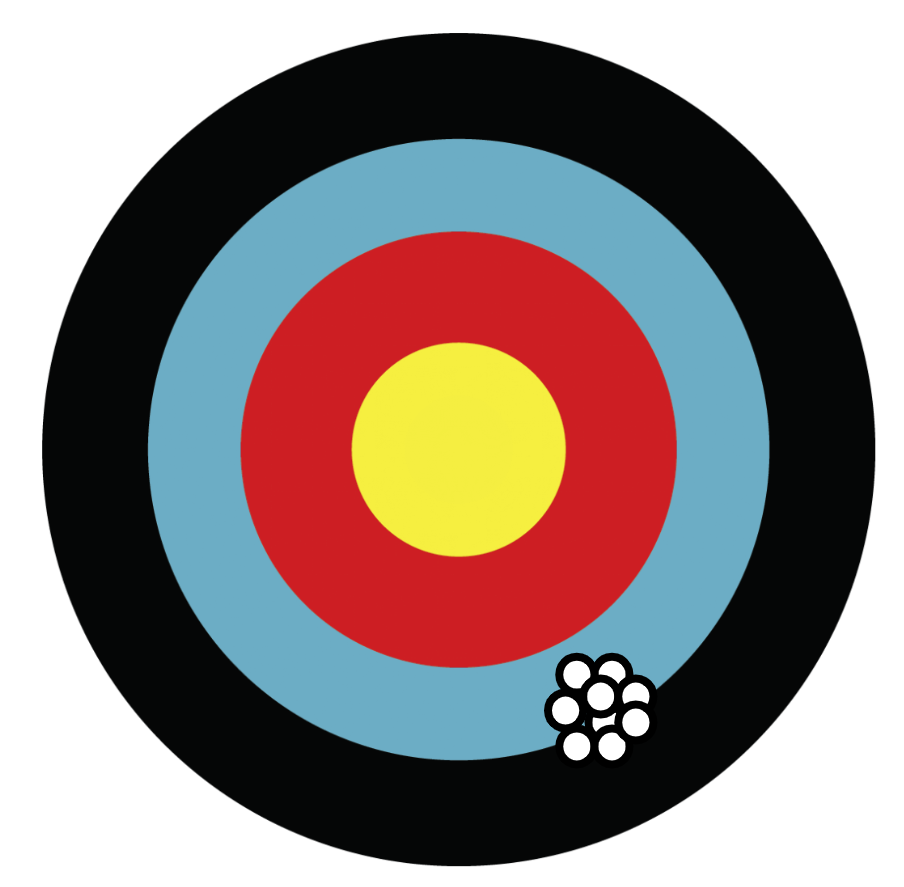
Biased outcomes are systematically off-the-mark however may nonetheless be tightly clustered collectively if variance is low.
In statistics, bias is all about systematic lopsidedness, whereas variance measures how unfold out the info are.
Variance is about having extra unfold within the knowledge. Excessive variance with out bias is a jolly type of incompetence that’s unfold out pretty. Which is why you do not wish to be standing inside 20 yards of me in any course once I play darts. It’s sufficient to make you would like I had been biased (within the normal course of the dartboard).
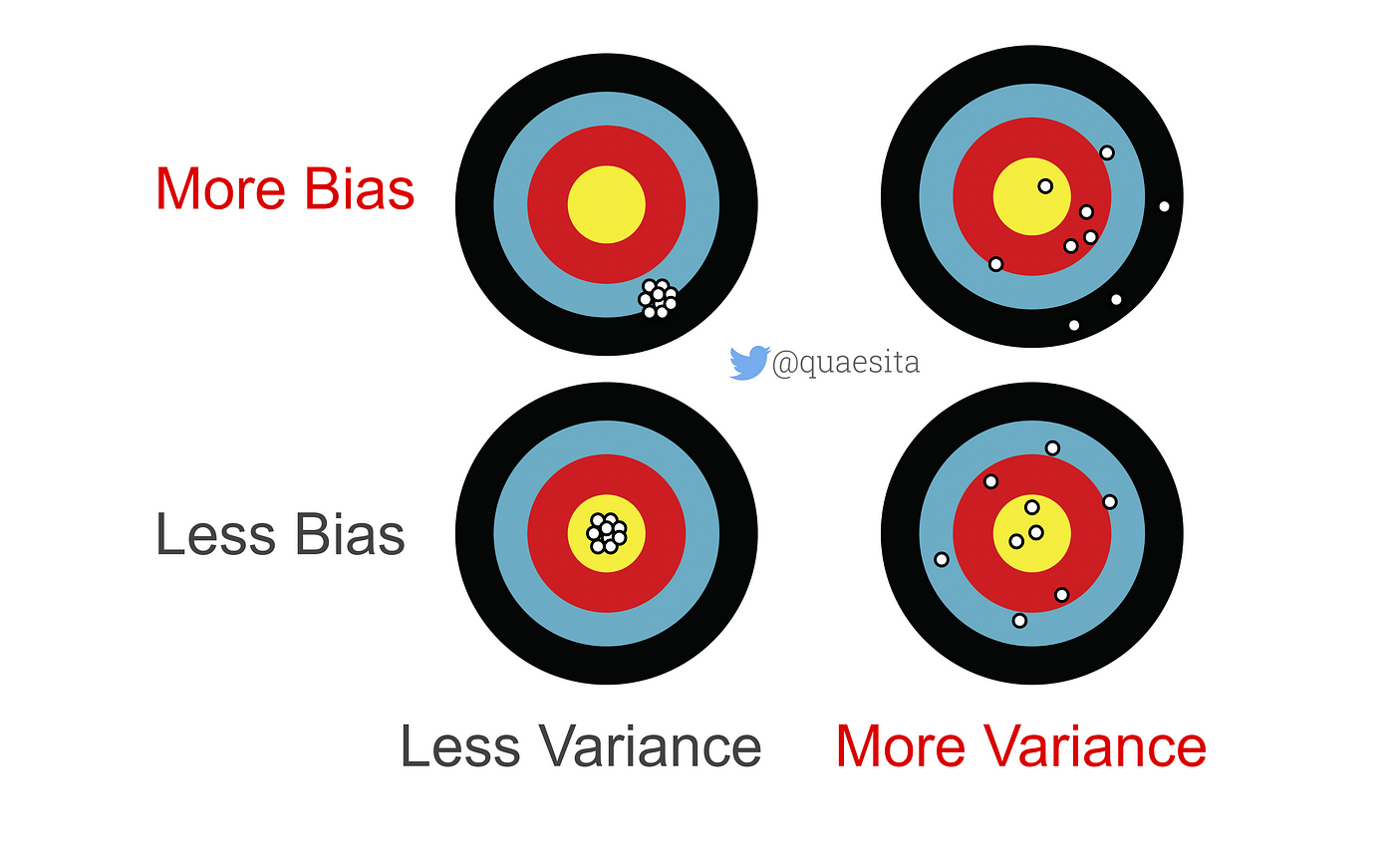
As you’ll be able to see from this diagram, the worst potential outcomes are these with excessive variance and excessive bias, whereas the very best combo is the one which retains them each low.
In case you arrived right here since you’re interested in an idea referred to as the bias-variance tradeoff in machine studying, an important factor to know is that the very best mannequin has no bias and no variance. In case you’re coaching a machine studying mannequin and also you handle to get each your bias and your variance to go down, there’s no want to speak about tradeoffs: when you grew to become a greater archer, you grew to become a greater archer. (It most likely took extra follow — knowledge! — to get there.)
However what when you couldn’t enhance one with out ruining the opposite? What if tightening your photographs meant being biased off course? And why would that occur? Why is that this essential? And why do most individuals who suppose they perceive the bias-variance tradeoff truly misunderstand it utterly?
I’ll reply these questions and extra on this mini-series on bias and variance — assist your self to that observe button so that you don’t miss out.

In case you’ve had your fill of variance, I recommend stepping over to my parallel article that makes use of a coronavirus case examine to show you about different kinds of bias: sampling bias, choice bias, info bias, reporting bias, and affirmation bias.
A few of you could want to go deeper, particularly when you’re eager to know the bias-variance tradeoff. In that case, keep tuned for the remainder of the sequence… although it’s about to get technical. However I’ve bought your again. Whilst you watch for the subsequent installment, you’ll be able to decide up all of the background you want with a view to make sense of it by following hyperlinks to my light intros.
Questioning when you ought to skip the background studying? Right here’s a guidelines of core ideas that I’ll be assuming you perceive in the remainder of the sequence:
- Inhabitants
- Pattern
- Statement
- Parameter
- Statistic
- Estimate
- Estimator
- Estimand
If any of them are unfamiliar, right here’s my useful vocab information that will help you out.
In case you’re curious in regards to the bias-variance tradeoff in machine studying — which is what this miniseries is constructing as much as — it’ll assist to have a passing familiarity with these fundamentals of ML/AI, which I cowl right here:
- What the purpose of ML/AI is
- Fashions
- Loss capabilities
As well as, you must know the definitions of every of those, which I’ve defined right here and right here:
- Anticipated worth
- Random variable
- Distribution
- Likelihood
- Imply
- Variance
Don’t fear! These ideas are a lot simpler than most individuals suppose and my guides take you thru them gently. Pleased studying!